News
Makin' Maps with Deep Learning
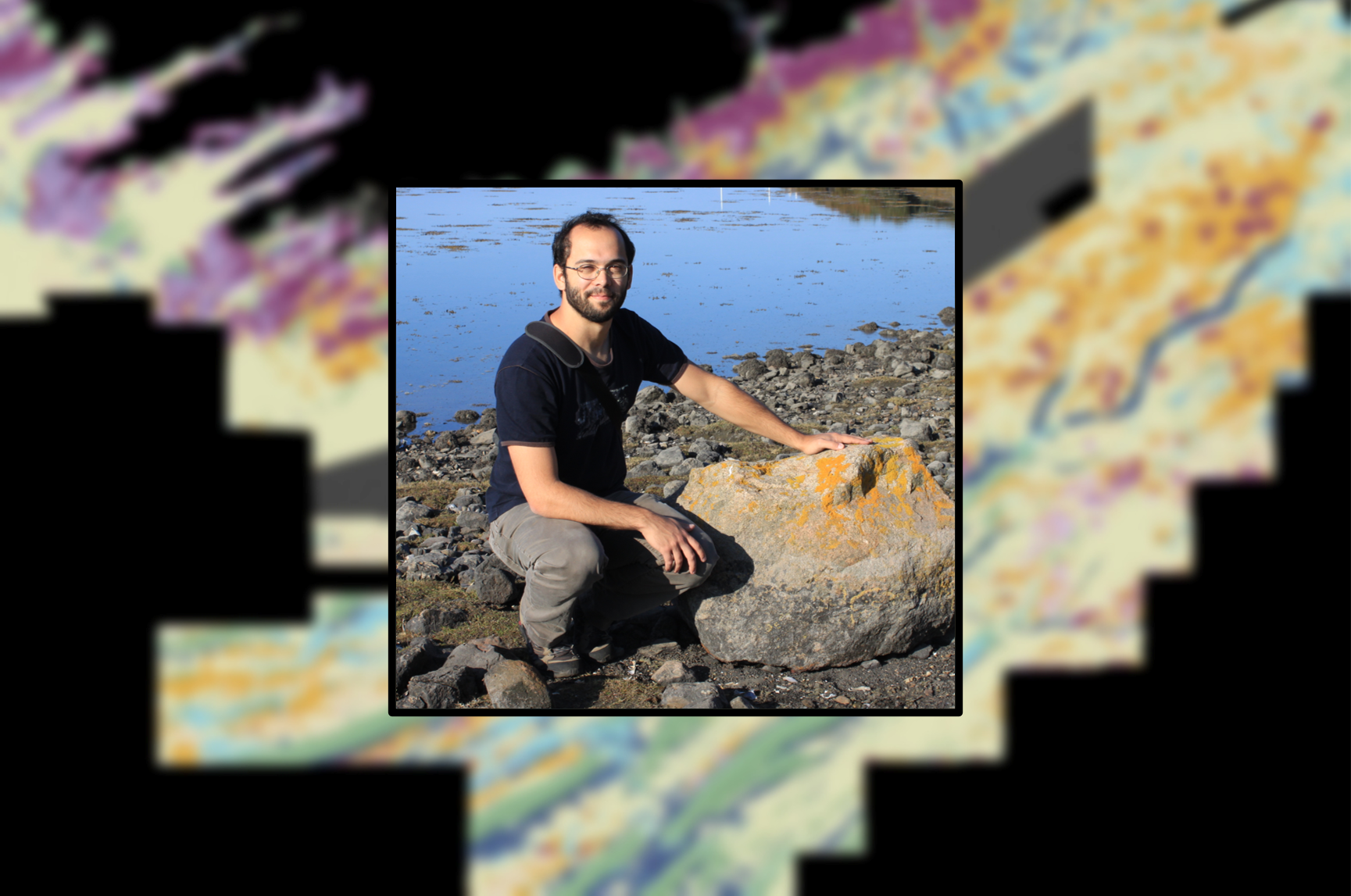
Dr. Riccardo Arosio has been busy with his project, NoMans_Tif
Deep Learning is a branch of artificial intelligence based on the idea that systems can learn from data, identify patterns and make decisions with minimal human intervention; mimic human understanding – if you will. In particular, Convolutional Neural Networks (CNNs) are an emerging set of algorithms within Deep Learning that produce pixel-wise classifications in order to create semantically segmented maps. The user has only to create a set of samples (“labels” in the jargon of the computer scientist) representing the classes that are to be identified. Labels and a dataset (e.g. imagery) are fed to the model and the model is trained to recognise the classes and to predict when they occur across the entire dataset.
CNNs are encroaching in many aspects of the scientific endeavour, for example they are used in the terrestrial and marine ecology community to map the extent of vegetation classes and sediment distribution. However, when it comes to geomorphology they are still uncommon and their application on elevation data in general (to recognise terrain shapes) is limited.
Brandon Hobley (University of East Anglia, the computer magician) and I have been working on the application of these CNNs on geomorphological mapping, trying to understand how best they can be applied in this science. We have tested various Fully CNN architectures (e.g. VGG13, 16 and Resnet50) on a set of common terrain derivatives (mainly obtained from the standard Benthic Terrain Modeller toolbox) of the seabed in the Celtic Sea. We have tried to simplify the task for the machine, creating a list of semantic classes that would: encapsulate all types of morphologies at seabed, be sufficiently distinct from one another, be morphologically simple. We ended up with a list of 10 classes.
As the results of the modelling come in, the potential of CNNs is obvious – see the figure below for a taste of a VGG13 prediction in the Celtic Sea. However the confidence is still far from perfect (accuracies of about 75-76%), more work is still needed.
We at the Marine Geoscience group in UCC can breathe a sigh of relief, machines have not stolen our jobs… yet ;)
Riccardo