Research News
Shane Galvin presented his PhD work at the New Advances in Online Research Methods: Virtual Summer School Conference at UCD
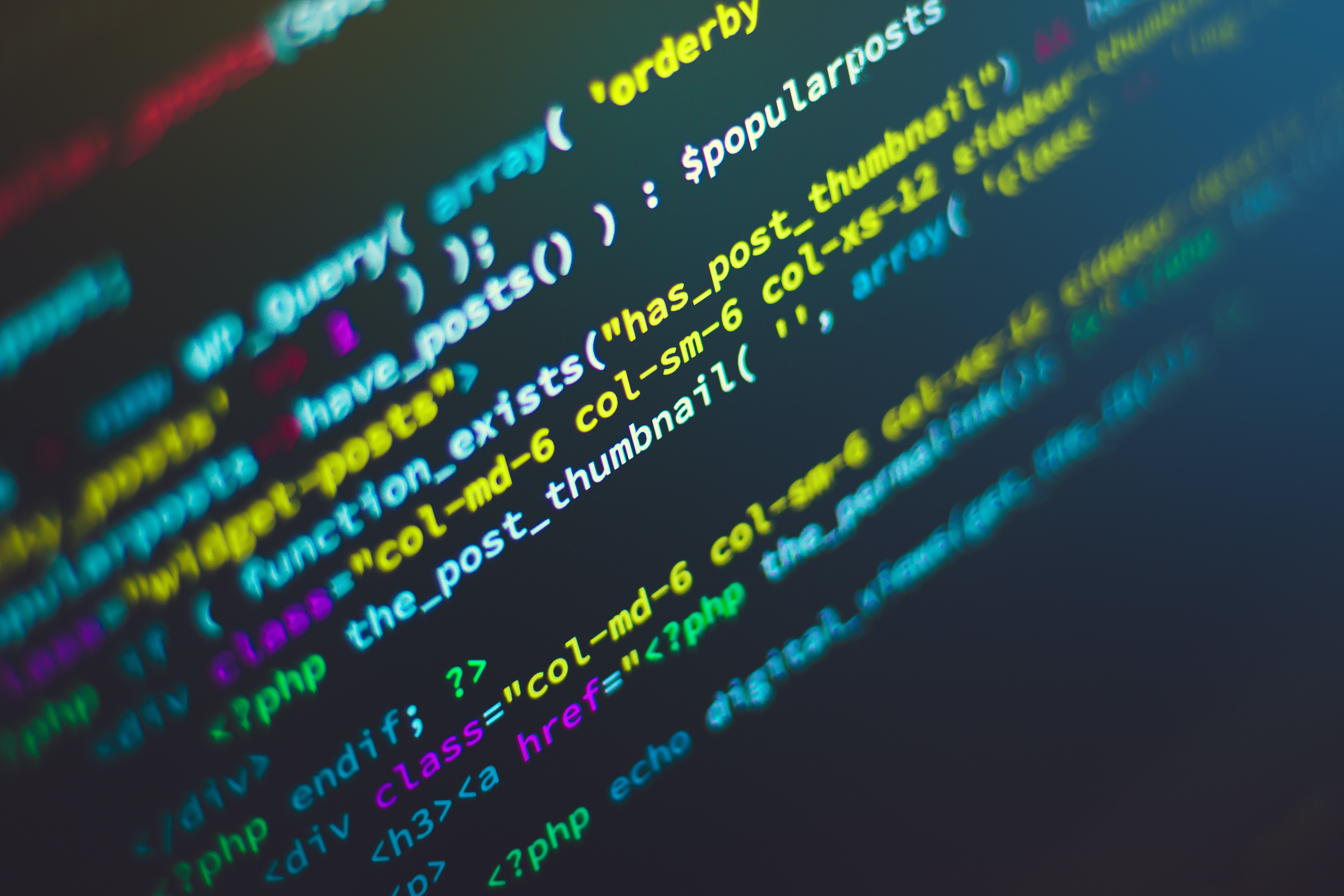
Shane Galvin and Raegan Murphy recently presented a poster on Shane's PhD work at the New Advances in Online Research Methods: Virtual Summer School Conference at UCD. Shane presented his work on his Rasch Analysis of the Matrix Reasoning Task. Data for the Matrix Reasoning Item Bank fits the dichotomous Rasch model. This may be useful to further internet-based research aiming to include measures of matrix reasoning, and to further the development of psychometric tools for use in internet-based research.
The ubiquity of internet based research, and prevalence of open science research methods signifies a need for an open use, psychometrically calibrated matrix reasoning test with (1) clear usage procedures, and (2) well understood measurement properties which may help to allay frequently observed measurement issues in psychology (see Flake & Fried (2020)). In particular, scale shortening can be supported using computerised adaptive testing (CAT) administration, e.g. Harrison et al. (2017). As an alternative to a large CAT item bank or parallel test forms, Explanatory Item Response Models (EIRM) (De Boeck & Wilson, 2004) allow for the automatic writing of Rasch compliant items (Gierl & Haladyna, 2012). This would assist with deployment of procedures in internet based testing with less concern for test security and scale validity threats. However, extension into advanced modeling and test administration requires the fitting of a measurement model prior to explanatory modeling. The present study is a step within a larger project that aims to detail the measurement properties and task structure of the Matrix Reasoning Item Bank (MaRs-IB) (Chierchia et al., 2019); a free-to-use, automatically generated bank of matrix reasoning items. This step aims to assess the psychometric properties of the MaRs-IB via Rasch analysis (Andrich, 1988; Rasch, 1960) to enable further validation research using EIRMs and extension into applied domains.
Participants: 485 participants completed an online procedure containing several demographic variables, a digits span forwards test, and a selection of 45 MaRs-IB items. Of the 485 participants, 443 participants remain; with 42 participants removed due to inappropriate response patterns, person misfit, or 0% / 100% score rate.
Design: The first 5 MaRs items were administered as practice items, with the remaining items presented in a pseudo-randomised order. Items were presented alongside 4 multiple choice response options, where the graphical position of each response options were also randomised. Items were delivered with a 30 second item-wise time limit. Of the 40 MaRs items scheduled for Rasch analysis (excluding practice items) 19 items remain. Rasch Analysis was conducted using Conditional Maximum Likelihood (CML) estimation as this conditions out the effect of the person ability distribution from item parameter estimation, and most closely represents the idea that the raw score should be a sufficient statistic for scaling items (Fischer & Molenaar, 2012).
Three comparison variables; device type (mobile vs desktop), biological sex (Female vs Male), and age group (age , age ); were used for conducting goodness of fit via Andersen’s likelihood Ratio Test (Andersen, 1973) (LRT), and differential item functioning (DIF) using a Wald Z test. Analysis of the relative fit of the dRM against a 2 parameter Item Response Theory (2pl IRT) model was also conducted. Bayesian Information Criterion (BIC) and Akaike’s Information Criterion (AIC) where used to identify which model possessed a better fit to the data, where lower relative values indicate better fit.
Software: Stimuli were presented using jsPsych (De Leeuw, 2015) (v 6.1.0). The procedure was administered to participants over the internet, who then used their own device and web browser. Statistical analysis was conducted using R (R Core Team, 2021), and Rasch Analysis via CML was conducted using the eRm package (Mair & Hatzinger, 2007) (v 1.0-2). Rasch and 2pl IRT models were estimated via Marginal MAximum Likelihood (MML) estimation using the TAM package (Robitzsch et al., 2021) (v 3.6-45).
For more on this story contact:
Raegan Murphy
raegan.murphy@ucc.ie